Written by Eva Everloo (Senior Analyst) together with Anđela Martinović, Ph.D. (Scientific Analyst), Anina Troya (Analyst Intern) & Orly Savion, Ph.D. (CTO)
PeakBridge
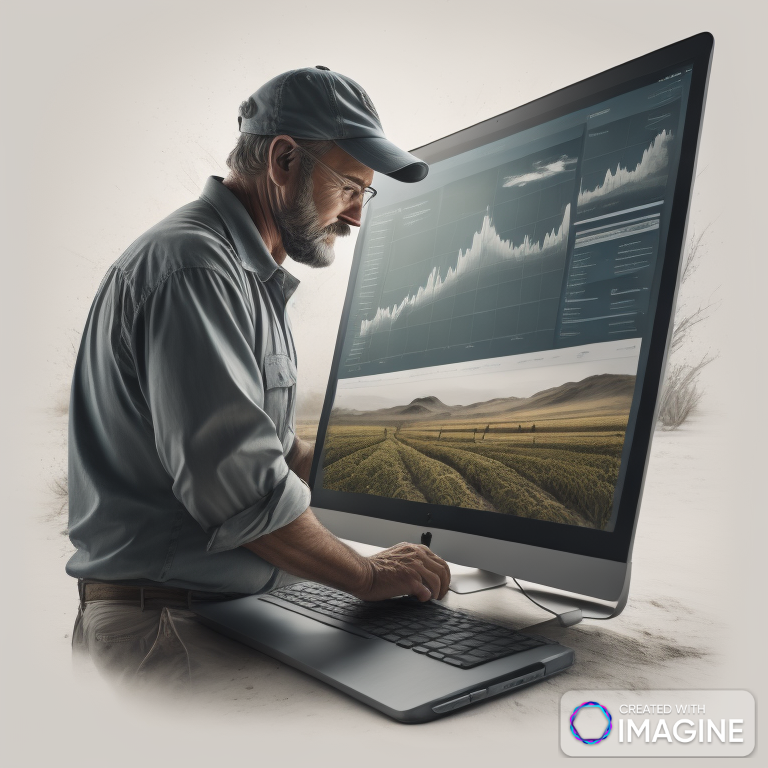
1. The Contemporary Globalized Food Value Chain
In today’s world, the global food value chain is like a complex puzzle that spans the planet, enabling the distribution of vital sustenance. This system involves numerous stakeholders, including farmers, manufacturers, distributors, retailers, and consumers, all interconnected in a delicate balance. In the 2020s, the world witnessed the disruption of this intricate network with the COVID-19 pandemic. Another more recent example is the Avian Flu outbreak[i] in the United States, which resulted in economic costs ranging from US$2.5 billion to US$3 billion, food shortages and exacerbated food prices. Imagine artificial intelligence (AI) and blockchain decentralized tracking networks had been in place to collect infectious disease data transparently and reliably[ii] and to forecast supply and demand with an optimized food network distribution.
Climate change is another challenge that impacts the entire food value chain, revealing its vulnerabilities. It has caused erratic crop yields, resulting in manufacturing shortages, increased transportation costs, and pricing fluctuations. This presents further challenges within the innovation agenda as procurement teams struggle to find sufficient materials meeting specific quality requirements, forcing them to use alternative materials that could affect the final product’s performance, ultimately influencing consumer purchasing behavior. The image below illustrates the current globalized food value chain and the complex, interdependent challenges faced by its stakeholders in today’s world.
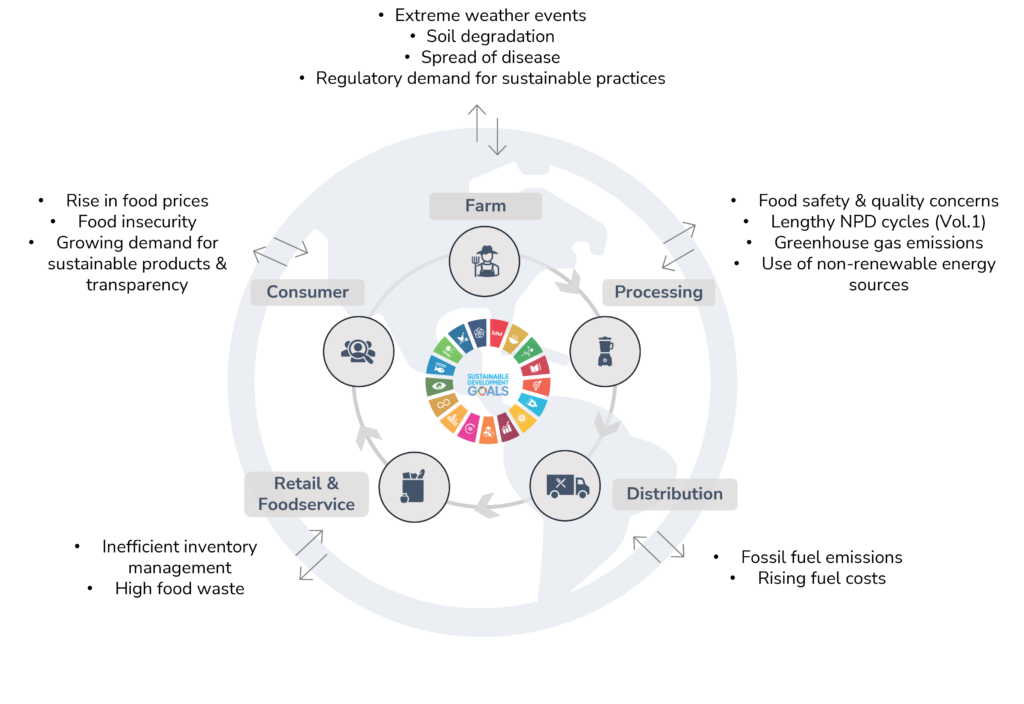
Beyond the immediate impacts of climate change and other external disruptions, the food value chain faces a host of other challenges including inefficient resource usage, operational inefficiencies, pest and disease monitoring issues, regulatory compliance demands, transparency expectations from consumers, food waste problems, sustainability concerns, difficulties in assessing environmental impact, slow innovation cycles, evolving consumer preferences, and infrastructure and technology gaps in certain regions.
These challenges require innovative technologies to support them. To improve the efficiency of the global food value chain, it is crucial to have quick communication and access to valuable information through a robust data network (alongside shared goals and alignment on KPIs across the value chain!). AI, through its capabilities in data analysis, predictive analytics, automation, and real-time monitoring, emerges as a transformative force in the industry that empowers the creation of a highly efficient, interconnected, and intelligent value chain, facilitating exceptional operational performance, now and in the future.
This article, following our previous exploration of AI’s role in new product development, delves into how AI is reshaping the food industry and its critical value chain (see Figure below).
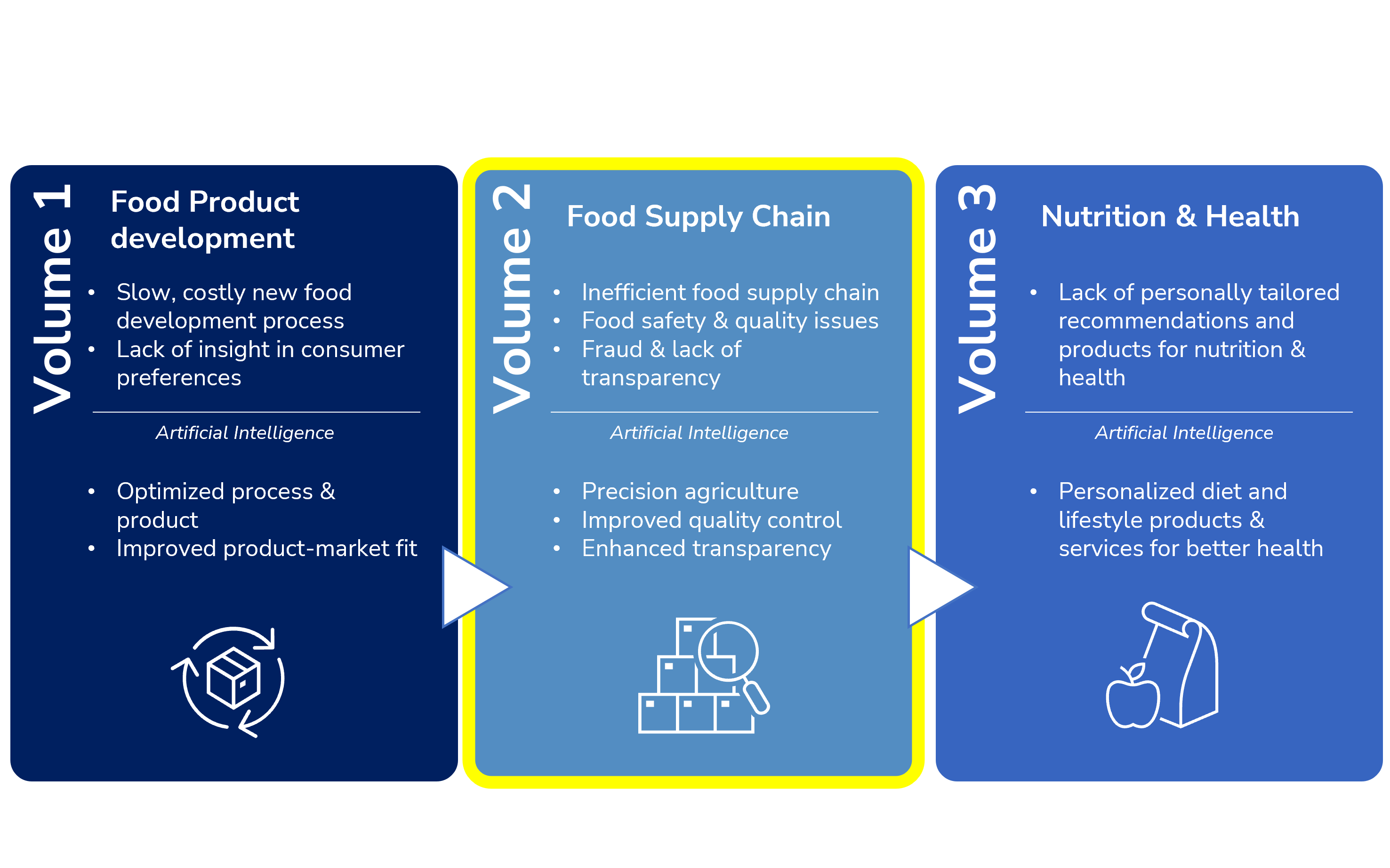
2. The Role of AI in Optimizing the Food Value Chain
2.1 Agriculture
Global agriculture faces challenges like manual labor, fertilizer, pesticide, and herbicide overuse, inaccurate demand predictions, resource inefficiency, crop diseases, and unpredictable weather, causing production variations affecting food supply. A major issue is 800 million small-scale farmers lack shared information platform, hindering crop decisions.
AI in Precision Agriculture:
- Real-time monitoring: AI provides real-time insights into critical factors such as soil moisture, crop water requirements, and weather predictions for precise decision-making. Another important application is the monitoring of biodiversity, including bee health and pollination efforts.
- Remote Sensing: AI-powered drones and satellites for crop health monitoring and yield estimation.
- Resource Optimization: AI algorithms optimize the use of fertilizers and pesticides, reducing waste and environmental impact.
- Smart Irrigation: Automated watering processes for water conservation.
- Robotic Farming: Autonomous AI-driven systems handle tasks like planting, harvesting, and weeding, improving efficiency and reducing labor costs.
Furthermore, AI is revolutionizing controlled indoor farming. It offers all-season production, reduces land use, and minimizes the need for chemicals. Here, AI aids to monitor and optimize environmental conditions for higher yields and resource efficiency (water / electricity / heat). Optimal control over growing conditions, including LED lighting type, color, and duration, can furthermore enhance food quality. These indoor farms are often located closer to consumers, thereby shortening food value chains, and reducing transport-related emissions. Despite the ongoing energy challenges, the integration of urban farming with AI presents a promising solution for ensuring food security and sustainability in densely populated regions with limited available farmland.
In livestock farming, AI-powered sensors have been developed to track the livestock’s state and behavior, allowing the detection of illness that are beyond human monitoring capabilities, enabling the efficient antibiotics administration on a large scale, minimizing the need for their excessive use, and curbing antibiotic resistance.
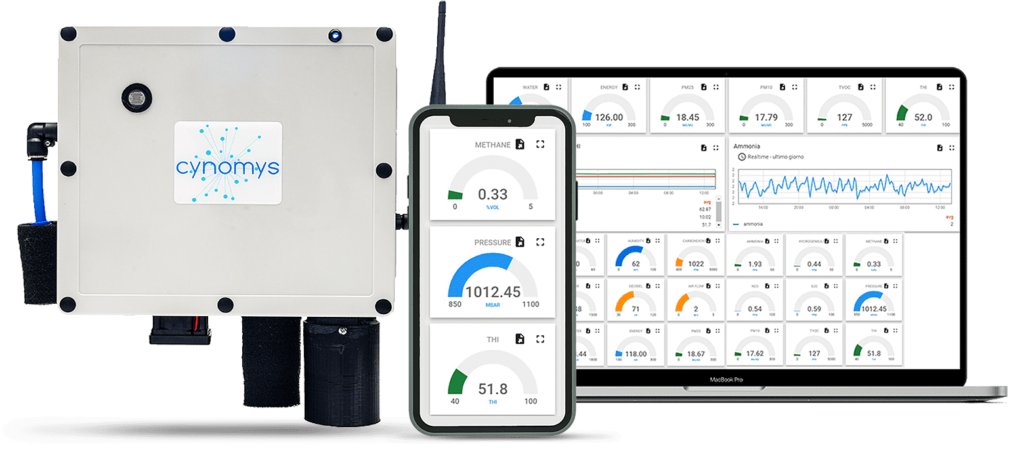
Startups:
- Hyperplan (FR) uses satellite data, weather, and AI to enhance crop production forecasting, economic efficiency, and greenhouse gas reduction. They collaborate with cereal group Vivescia and Corteva Agriscience, specialized in seeds and crop protection[iii].
- US-based Leaf (US) provides a unified data infrastructure that consolidates data from various sources (satellite, weather, machines, drone field imagery, etc.) that they sell to companies with existing data architecture that serve farmers.
- Blue Circle (IL) predicts grape ripening using AI and historical weather data.
- Tortuna AgTech (US) employs robotics, data, and AI for harvesting, forecasting, and pest control in agriculture.
- AgEye (US) offers “plant scientist-as-a-service” using computer vision and deep learning algorithms to monitor crop growth, enhancing operational efficiency in controlled indoor farming.
- 80 Acres Farms (US) operates fully optimized indoor vertical farms with automated monitoring and control and robots. These farms can produce up to 300 times more food than an ordinary farm with 100% renewable energy and 95% less water.
- BeeHero (US) has developed IoT sensors that transform conventional beehives into intelligent, smart hives with the capacity to tackle the worldwide pollination shortage.
- Cynomys (IT) monitors over 30 environmental parameters including fine dust, ammonia, hydrogen sulfide, and methane to increase environmental sustainability and reduce livestock mortality.
The field of precision agriculture is experiencing a surge in partnerships between established industry players and startups[iv], driving innovation and scale. For instance, in 2023, 80 Acres Farms partnered with Siemens Technology to support expansion to meet global food demands[v] solutions. One thousand Kroger stores are already being supplied with their leafy vegetables, micro greens, and herbs[vi]. In 2021, US irrigation equipment and services company Valmont Industries acquired Israeli startup Prospera Technologies that leverages AI and computer vision technologies to enhance irrigation and other agricultural practices[vii]. The European Space Agency (ESA) has joined forces with Delft University of Technology in the Netherlands to create the Agricultural Sandbox, making satellite data accessible to agriculture professionals[viii]. France-based Hiphen (image analysis specialist) furthermore acquired California-based SlantRange, a provider of drone-based crop analysis and precision agriculture data. This strategic move strengthens Hiphen’s position in the precision agriculture sector[ix]. Additionally, Hummingbird Technologies partnered with Agreena, Europe’s leading carbon farming platform, to promote regenerative agriculture through remote sensing and precision agronomy tools[x]. These partnerships highlight the collective effort to leverage AI and innovative technologies for more sustainable and productive farming.
2.2. Quality Control & Distribution
After crops are harvested at the right maturity, they are transported from farms, to processing facilities, CAFO’s, wholesale storage distribution centers, retail outlets, restaurants, and ultimately, consumers. This distribution network faces challenges, ranging from inadequate storage, inventory management issues, to transportation problems, all contributing to high costs and food waste. Food quality control processes are time-consuming and prone to (human) error resulting in significant food waste (e.g., the need to discard an entire truckload of fresh produce). Efficient food storage, especially cold storage, is critical for maintaining food quality and safety. The rise of e-commerce and direct-to-consumer sales further emphasizes the importance of optimizing inventory management. Additionally, transportation and distribution involve issues like rising fuel costs, regulatory compliance, infrastructure limitations, and sustainability concerns, especially with the growing demand for just-in-time deliveries and the expansion of last-mile logistics.
AI in QC & Distribution:
- Precise Inventory Forecasting: Accurate stock predictions, reducing overstocking and understocking.
- Energy Efficiency: Employing AI to optimize warehouse operations, including lighting, temperature control, and equipment usage, thereby reducing energy consumption and operational costs.
- Quality Control: Enhancing inspection accuracy through AI-driven automation.
- Waste Reduction: Implementing AI-driven strategies for improved inventory management, reducing the likelihood of food spoilage and waste.
- Predictive Maintenance: Using predictive maintenance models to anticipate equipment breakdowns in transportation preventing costly delays.
Startups:
- Israel-based Neolithics (IL) leverages hyperspectral image analysis and ML to detect, classify, and sort crops based on their condition on conveyors, addressing inconsistent quality control and product losses in distribution chains.
- Imperfect Foods (US) is using AI to connect consumers with groceries that are close to where they live and deliver imperfect foods in a convenient way to a customer’s doorsteps.
- Farmstead (US) is an AI-powered online grocery platform that helps restaurants, grocers and wholesalers predict demand, cut food waste, and reduce stockouts.
Examples of notable partnerships include Tyson Foods, a meat producer, that invested[xi] in Clear Labs, a startup specializing in DNA-based food testing. Clear Labs uses AI and DNA sequencing to provide food safety and quality assurance, which in the meat industry poses a critical public health risk[xii]. Furthermore, TeleSense (US) recently got acquired[xiii] by DECCO Post Harvest, a provider of grain storage and transportation monitoring technology using wireless sensors and advanced analytics. Lastly, Apeel Sciences acquired[xiv] ImpactVision in 2021, a ML company developer of a food security and quality software platform, applying hyperspectral imaging technology to food.
2.3 Sale: Retail + Foodservice
Challenges in the retail sector revolve around delivering efficient grocery shopping experiences, minimizing food waste, and optimizing inventory. A significant hurdle is the lack of integrated data, impacting inventory visibility, personalization, and overall value chain management. This hinders retailers from effectively understanding and engaging customers, refining pricing strategies, and adapting to evolving consumer behavior and market dynamics. Moreover, with the surge in e-commerce and contactless shopping, long-term competitiveness further highlights the importance of adopting integrated data solutions.
AI in Retail:
- Demand Forecasting & Inventory Management: AI-driven demand prediction models to gain insights into consumer demand, aiding in better stock management, reducing waste and operational costs as well.
- Personalized Shopping: Enhanced personalized shopping experiences based on behavioral data and historical purchases. Virtual shopping assistants contribute to this by providing recommendations and assistance.
- Pricing Strategy Refinement: Utilizing AI to refine pricing strategies through improved understanding of consumer behavior and market dynamics.
In the foodservice sector, challenges encompass streamlining kitchen operations for increased efficiency, maintaining rigorous food safety standards, optimizing menus, and pricing, and enhancing the overall dining experience to ensure customer satisfaction, build loyalty, and reach profitability.
AI in Foodservice:
- Improved Efficiency: Streamlining restaurant operations for enhanced efficiency and reduced food preparation time.
- Food Safety Compliance: AI-powered systems for rigorous monitoring and ensuring compliance with food safety regulations throughout the value chain.
- Customer Service Enhancement: Personalizing service, managing wait times, and efficiently processing orders to enhance overall dining experiences.
- Demand Forecasting: Accurately predicting customer traffic through historical and real-time data for better resource allocation.
- Menu Optimization: Optimizing menu offerings and pricing strategies to cater to diverse customer preferences while maximizing revenue.
- Staffing Solutions: AI-driven robots and automation to address staffing challenges and improve workforce efficiency in the foodservice industry[xv]. Challenges with this technology include the need for vast amounts of data, steep capital requirements and a long path to revenue.
Startups:
- Singapore-based Trax (SG) leverages AI to enhance shelf management and inventory tracking. Using computer vision and ML, they provide real-time insights into product availability, shopper engagement, optimizing in-store experiences.
- Shelf Engine (US) employs AI to help grocery stores optimize stock levels, reducing food waste and operational costs. This aligns with the growing trend of contactless ordering and payment options, making shopping more convenient for consumers.
- TasteWise (IL) provides intelligent consumer and market insights by tracking restaurant menu data alongside social media insights.
- Delicious Data (DE) focuses on QSR operations improvement, addressing food waste and other operational inefficiencies through AI-driven demand forecast models.
- Instacart (US) provides retailers with products and services to power their e-commerce experiences, fulfill orders, digitize brick-and-mortar stores, provide advertising services, and insights for improved consumer engagement and healthier choices.
NSYNQ3 Restaurant Solutions’ acquisition[xvi] of Novo Labs, specializing in conversational AI for high-volume voice ordering channels, and Square’s acquisition[xvii] of GoParrot in 2022 to provide an omnichannel software solution for restaurants and franchises, facilitating direct customer connections through an all-in-one digital ordering and marketing platform. Another interesting development is how Amazon leverages data resources and the AWS infrastructure to offer seamless online grocery shopping experiences with Amazon Go and Amazon Fresh[xviii].
2.4 Waste Management
The inefficiency in matching global food supply with demand leads to approximately one-third of the world’s food being wasted . Wasted food translates to squandered land, heightened emissions, and excessive water use, worsening climate issues that, in turn, disrupt the value chain. For food corporates, this means economic instability, decreased profits, and a volatile market. Excessive food waste, thus, is not only a pressing concern for the environment, and for food security of the growing global population, but also for the business bottom line. Waste minimization is a critical objective throughout the food value chain that requires collaboration among global stakeholders.
AI in Waste Management:
- Data-Driven Supply Optimization: AI utilizes historical and real-time datasets for efficient production and distribution, ensuring that supply aligns with consumer needs, minimizing overproduction, reducing excess inventory, spoilage and minimizing waste.
- AI Applications for Enhanced Recycling: machine learning classification algorithms identify waste types like plastics, metals, and paper[xx].
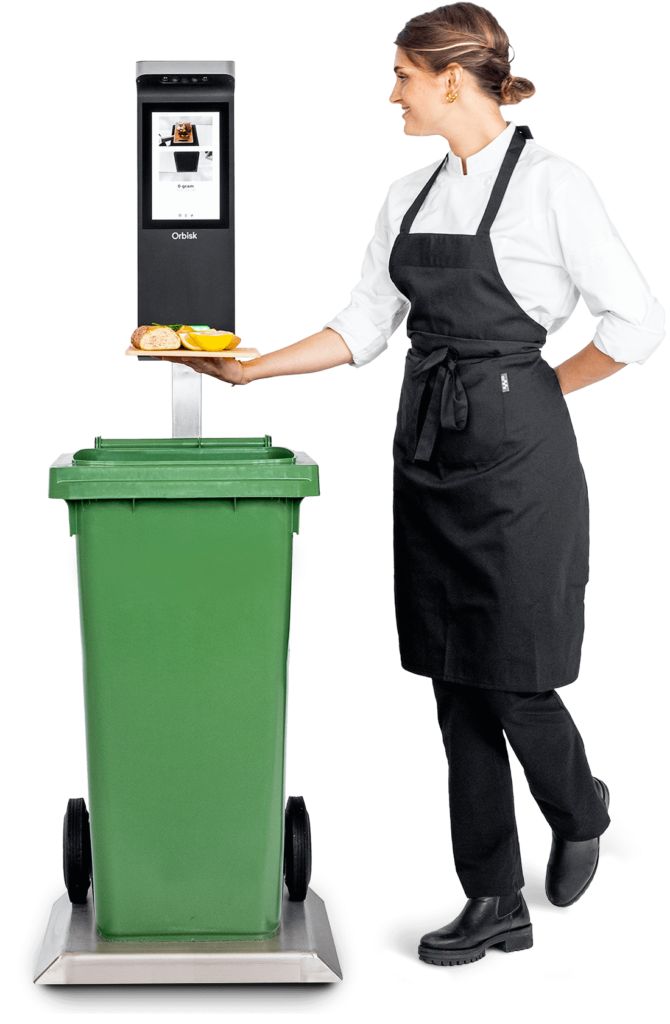
Startups:
- Orbisk (NL) provides professional kitchens with their fully automated food waste monitor to gain insight into their specific food waste.
- Prairie Robotics (CA) uses AI-powered cameras on organics and recycling trucks to detect household waste. By analyzing this data, the company sends highly personalized notifications to residents, which have been proven to markedly reduce waste levels.
A notable startup-industry partnership is the acquisition[xxi] of France-based CodaBene, a food waste management platform that supports retailers in digitizing their data and minimizing food waste, by Too Good to Go.
2.5 Transparency & Traceability
One of the prevailing challenges in the food value chain lies in the lack of transparency. Consumers today seek greater visibility into the origin, production method, and journey of food products. The significant surge, a 71% increase, in online searches for sustainable goods between 2016 and 2021[xxii] shows that provenance is a key growing consumer need that helps to build trust amongst the food industry. However, existing systems often fall short in providing comprehensive information, leading to concerns about misleading food labeling and conflicts of interest within the organizations responsible for these labels. Additionally, certification requirements at various stages of the value chain can drive up implementation costs, further complicating the quest for transparency.
AI in Transparency & Traceability:
- Blockchain Integration: Blockchain, known for its ability to maintain transparent and immutable transaction records, provides a robust tool for tracking a product through its stages in the value chain (in contrast to centralized labelling systems).
- Food Recall Enhancement: Blockchain enables the near-instantaneous identification and removal of contaminated food products.
- Product Traceability: Product-specific QR codes with detailed tracking information allow consumers to trace a product’s journey from farm to table.
These advancements mark a new era of transparency and accountability in the food value chain, aligning with the evolving demands of ethical and informed consumers.
Startups:
- Agriplace (NL) is an independent platform that creates smart tools to make certification easy and enables quick data sharing with auditors and buyers.
- RegAsk (SG) provides technology that routinely monitors, predicts, and reports updates or changes to relevant regulations, providing instant alerts.
- Index Biosystems (CA) is using biotechnology for traceability called BioTags – microscopic fingerprints that are applied directly to products enabling downstream verification of sustainability claims, quality assurance and food safety.
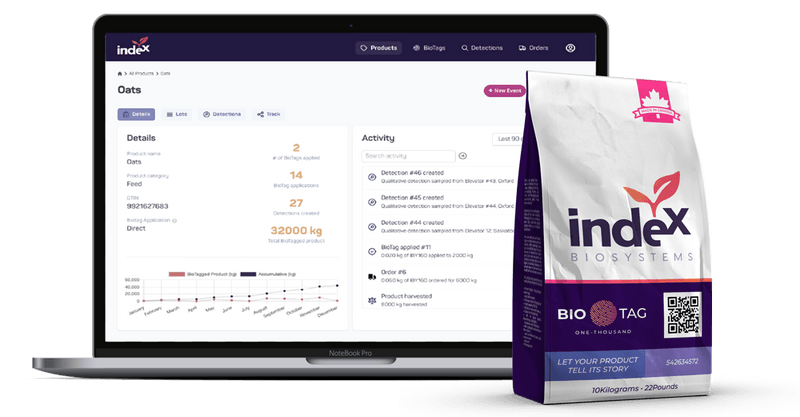
2.6 Impact Assessment
Environmental impact assessment is crucial for making informed decisions that promote sustainability, minimize harm to the planet, and ensure transparency, legal compliance, and long-term planning in the food value chain. However, today, it faces several challenges, including the lack of insight into emissions and standardized methods for measuring carbon footprints. This, combined with unclear regulatory guidance, leaves concerns about CO2, methane, and nitrous oxide emissions unaddressed and contributes to ambiguity for industry stakeholders. Determining responsibility for environmental impact in a globalized value chain is complex, particularly in the context of uncertain emissions’ effects on the global climate and the debate about whether responsibility lies with consumers or production countries. To progress, there is a pressing need for open-source carbon footprint data and standardized measurement frameworks. Additionally, reconfiguring subsidies, local legislation, and international trade agreements is crucial to realign economic incentives with sustainability goals.
AI in Impact Assessment:
- Measurement Reliability: AI enhances the reliability of environmental impact measurements by utilizing historical and real-time data.
- Standardized Measurement Frameworks: AI supports the development of standardized measurement frameworks across the food value chain, promoting consistency and comparability.
- Alignment with Regulations & Economic Incentives: AI helps align environmental impact assessments with regulatory requirements and economic incentives, facilitating responsible practices throughout the industry.
This transformation calls for collaborative efforts from regulators, innovators, and industry leaders, placing AI-driven technologies and startup initiatives with the important responsibility of developing the solutions that reshape our approach to environmental sustainability.
Startups:
- Carbon Cloud (DK) leverages AI to assess the climate footprint of food products to reduce negative climate impact. It turns decades of climate research into actionable insights for the food industry.
- Mondra (UK) is combining satellite data and AI to support food companies meeting their carbon footprint neutrality goals by tracking the environmental impact of food throughout the entire value chain.
Two notable partnerships are the acquisition[xxiii] by IBM of Envizi (AU) that provides AI-powered software to help organizations in creating more resilient and sustainable value chains by simplifying the capture, consolidation, management, analysis and reporting of their ESG data, and the collaboration, between Albo Climate and ALUS (Canadian NGO) for the use of remote sensing to model carbon and land use in wild and cultivated ecosystems[xxiv]
3. Startup Landscape – Food Supply Value Chain
A surge of startups dedicated to AI-driven solutions has emerged across the entire food value chain. They represent a dynamic landscape that revolutionizes the food industry by leveraging artificial intelligence to enhance sustainability, efficiency, and transparency, ultimately shaping a more sustainable and connected food value chain.
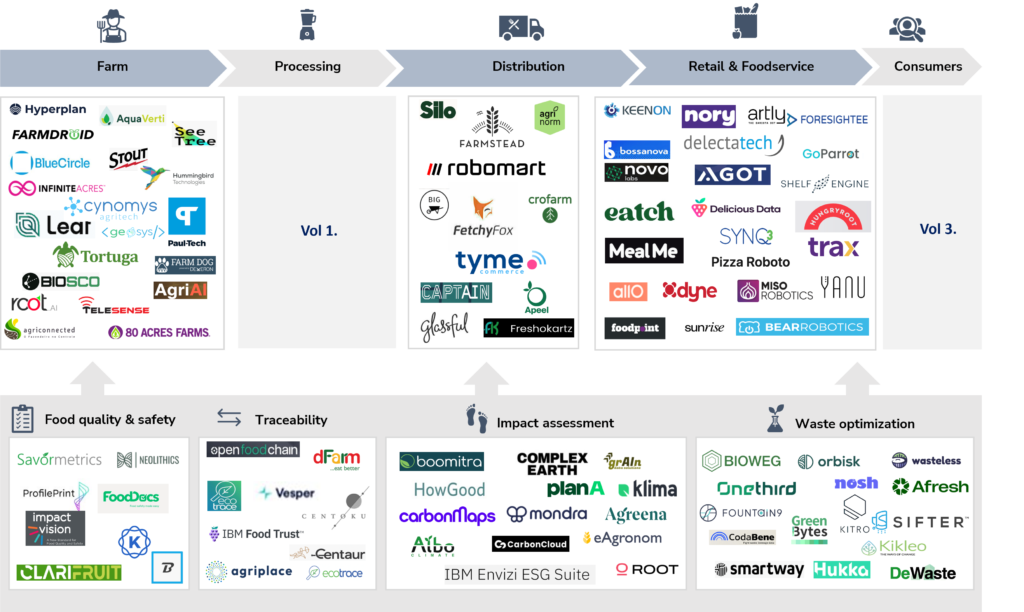
Disclaimer: this overview is not exhaustive and only represents a selection of startups. The categorization of companies is furthermore non-exclusive, meaning startups can fall under multiple categories.
4. Challenges & Risks for AI across the Food Value chain
In Volume 1 of this deep dive series, the general challenges for AI in food were outlined. Here, we dive deeper into the challenges and risks related specifically to the food value chain.
- Standardization & Fragmentation: inconsistent data stemming from varied collection methods and a lack of standardization and protocols hinders data sharing and interoperability. For environmental emissions data, the lack of clarity about the way it is calculated (e.g., CO2 impact of cattle feed in/out) adds further complexity.
- Data sharing & Integration: stakeholders utilize diverse systems and technologies, making data sharing complex. Incompatible software across farms, transportation companies, and retailers disrupts operations and requires organizational workflow changes for successful AI integration.
- Adaptation to Dynamic Fluctuations: AI systems must rapidly adapt to dynamic fluctuations caused by seasonality, trends, or unexpected events like natural disasters or pandemics, such as the challenges posed by the COVID-19 pandemic.
- Expertise Integration: while AI technology advances, it requires seamless integration into growers’ operations. Bridging the gap between technological innovation and agricultural expertise is crucial for its successful adoption.
- Resistance to Adoption: Farmers may be hesitant to embrace technology, potentially slowing down the adoption of AI in agriculture.
- Foodservice data: Comprehensive data on wholesale purchases and consumer sales are still lacking for foodservice, limiting the applications of AI in this sector today. Existing syndicated data providers predominantly serve the retail sector.
Addressing these challenges requires flexibility, operational alignment, and collaboration across the entire agri-food value chain.
5. Bottom Line: Paving the Path Ahead
The current state of the food industry is marked by numerous challenges and inefficiencies that hinder its ability to meet the demands of a growing global population. From disjointed value chain stages to inadequate data-sharing mechanisms, the industry grapples with complexities leading to waste, inefficiencies, and a lack of transparency. These challenges span the entire food value chain, from agriculture to quality control, distribution, retail, foodservice, waste management, and transparency, adapting to consumer preferences.
Amid these challenges lies an opportunity for transformation through AI. AI applications in farming are generating vast amounts of data that can bridge these gaps. By leveraging AI to integrate data across the value chain and establishing a reliable connectivity infrastructure, the industry can align its operations, facilitate coordinated planning, and foster collaborative relationships across the entire spectrum, from Farm to Fork. AI is already revolutionizing different segments of the food value chain, including improved efficiency, transparency and food quality, reduced waste, and increasing sustainability. In this transition, startups are playing a vital role in driving innovation and collaboration with established industry players.
However, this vision for a more interconnected and intelligent agri-food value chain also requires a broader collaboration and aligned strategies among all stakeholders. Other conditions that need to be met to unlock the full transformative potential of AI include the standardization, sharing and integration of data.
As we look ahead to Volume 3, which explores AI in health and nutrition, we see a promising future where AI continues to shape a more interconnected and intelligent agri-food value chain.
Further Reading
- Artificial Intelligence in Extended Agri-Food Value chain: A Short Review Based on Bibliometric Analysis
- Artificial Intelligence in Food Safety: A Decade Review and Bibliometric Analysis
- Technology and Connectivity Can Help Improve Our Food Supply
- Emerging trends in the agri-food sector: Digitalisation and shift to plant-based diets
[i] https://www.fairr.org/resources/reports/industry-reinfected-avian-flu
[ii] https://www.ncbi.nlm.nih.gov/pmc/articles/PMC9187510/
[iii] https://www.lefigaro.fr/economie/hyperplan-la-plateforme-collaborative-de-suivi-des-cultures-pour-securiser-la-chaine-agro-alimentaire-20230220
[iv] https://agfundernews.com/increasing-agtech-partnerships-a-precursor-to-consolidation-in-precision-ag5564
[v] https://press.siemens.com/global/en/pressrelease/siemens-and-80-acres-collaborate-scale-vertical-farming
[vi] https://ir.kroger.com/CorporateProfile/press-releases/press-release/2023/Kroger-and-80-Acres-Farms-to-Reach-More-Customers/default.aspx
[vii] https://agfundernews.com/prospera-valmont-acquires-crop-analytics-startup-for-300m
[viii] https://www.esa.int/Applications/Observing_the_Earth/Copernicus/Sentinel-1/Monitoring_crop_health_across_the_Netherlands
[ix] https://www.finsmes.com/2023/06/hiphen-acquires-slantrange.html#:~:text=Hiphen%2C%20an%20Avignon%2C%20France%2D,a%20platform%20for%20drone%20phenotyping.
[x] https://agfundernews.com/agreena-acquires-hummingbird-technologies-to-strengthen-the-integrity-of-carbon-farming
[xi] https://www.clearlabs.com/tyson-ventures-invests-in-clear-labs-on-a-mission-to-safely-feed-a-growing-world-population/
[xii] https://www.reuters.com/business/healthcare-pharmaceuticals/usda-reports-highly-lethal-bird-flu-kentucky-chicken-farm-2022-02-14/
[xiii] https://news.agropages.com/News/NewsDetail—44037.htm
[xiv] https://agfundernews.com/impactvision-acquired-by-apeel-to-look-inside-fruit-veg-for-freshness
[xv] https://www.forbes.com/sites/markcperna/2022/07/05/why-americas-restaurant-staffing-crisis-could-make-it-harder-to-go-out-to-eat/?sh=5976e4cb2653
[xvi] https://dallasinnovates.com/dallas-based-conversational-ai-service-novo-labs-acquired-by-synq3-restaurant-services/
[xvii] https://squareup.com/us/en/press/square-acquires-goparrot
[xviii] https://www.pocket-lint.com/nl-nl/gadgets/nieuws/amazon/139650-wat-is-amazon-go-waar-is-het-en-hoe-werkt-het/
[xx] https://www.sciencedirect.com/science/article/abs/pii/S095965262301716X
[xxi] https://www.toogoodtogo.com/en/toogoodtogo-acquires-codabene
[xxii] https://www.worldwildlife.org/publications/an-eco-wakening-measuring-awareness-engagement-and-action-for-nature
[xxiii] https://www.techtarget.com/searchbusinessanalytics/news/252511936/IBM-unveils-acquisition-of-Envizi-to-add-ESG-analytics-tools#:~:text=IBM%20revealed%20that%20it%20completed,the%20deal%20were%20not%20disclosed
[xxiv] https://www.albosys.com/post/albo-climate-and-alus-partner-to-leverage-remote-sensing-and-machine-learning-for-new-acre-project